Cracking the code of Arctic sea ice leads
Cracks or fractures in the Arctic sea ice, known as leads, leave lines of open water. Scientists are increasingly looking at sea ice leads to determine whether their presence is related to diminishing ice in the Arctic and to learn more about ocean-atmosphere dynamics. Answering these questions, however, requires reliable data and methods for detecting them.
Using satellite instruments to detect and study sea ice leads is not new, but what has changed dramatically are the sensor technologies and improved resolutions that provide more and better detail. Taking advantage of those improvements, researchers at the University of Wisconsin–Madison Cooperative Institute for Meteorological Satellite Studies (CIMSS) have developed an updated algorithm to detect and characterize sea ice leads and to analyze trends.
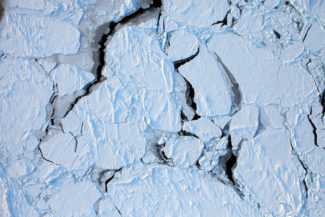
Arctic sea ice. Credit: NASA
Principal Investigator Steve Ackerman wanted to take a new look at sea ice leads using Moderate Resolution Imaging Spectroradiometer (MODIS) data. He partnered with Jeff Key, a scientist with the NOAA Advanced Satellite Products Branch (ASPB) stationed at CIMSS, who has unique experience using Landsat data to study Arctic sea ice leads. Rounding out the collaboration are Yinghui Liu, another NOAA ASPB scientist stationed at CIMSS with knowledge of polar region retrievals, and Jay Hoffman, the CIMSS researcher who developed the new algorithm.
Their updated method begins with a number of tests (cloud mask, ocean mask, and thermal contrast) of the MODIS data to eliminate areas that do not contain leads. Once leads are initially detected, additional tests (e.g., shape, width, and symmetry) are applied to verify the initial detection or further eliminate any areas that have been falsely identified as containing leads. A Hough Transform looks for linear features in the data. The last step is to characterize any detected leads in terms of length, width, area, and orientation. The output of the sea ice leads algorithm, in the form of NetCDF files, is available on their website: ssec.wisc.edu/leads.
The researchers placed two constraints on the MODIS data used in the algorithm, one to manage clouds and the second to maximize the benefits of two MODIS sensors flying simultaneously. Because of clouds, only data from January through April are currently considered, though the team is discussing the possibility of expanding that to November through April. Any further attempts to broaden the algorithm to include other months would face significant issues in the form of increased cloud cover and the lack of temperature differences between sea ice and water or thin ice in the leads. As Hoffman notes, “the algorithm works fundamentally by temperature contrast.”
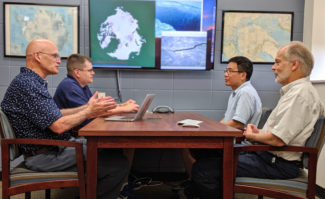
The Arctic sea ice leads team at CIMSS (from left to right: Steve Ackerman, Jay Hoffman, Yinghui Liu, and Jeff Key) has developed an improved algorithm to detect and characterize sea ice leads using MODIS data and is adapting the algorithm to use higher resolution VIIRS data. Credit: Eric Verbeten
In addition, while the first MODIS instrument was launched on the Terra satellite in 1999, the algorithm is applied to MODIS data only as far back as 2003. That was the first year in which two MODIS instruments were in orbit — with a second one flying on the Aqua satellite — allowing for multiple overpasses and observations of the same features over time.
“That’s one of the advantages of having a two-satellite system — typically, upward of 30 overpasses everywhere in the Arctic [per day]. You want to detect a lead feature at the same location multiple times to have some confidence that it is actually a lead, rather than clouds that have similar thermal contrast signatures but tend to be much less stationary,” says Hoffman.
The new algorithm development builds on Key’s work on sea ice leads in the early 1990s. While Key describes his efforts as “a proof of concept,” Ackerman notes that “it really laid the foundation of how we thought about detection.” At their core, both algorithms were designed to make the best use of available satellite data, given the state of sensor technology at the time.
“The goal is the same, which is to detect leads in satellite imagery and characterize those leads in terms of their width, distribution, their orientations, length and area,” says Key.
With their success in developing the algorithm to use MODIS data, the team received a second grant from NASA to adapt it to work with Visible Infrared Imaging Radiometer Suite (VIIRS) data. One challenge is the difference in resolution between the two sensors. In contrast to MODIS, VIIRS has two different 11 micron channels — the channel used in sea ice lead detection — with two different resolutions, notes Hoffman. In addition, the higher resolution did not automatically translate to improved detection of more leads.
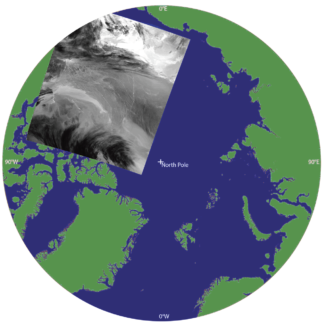
MODIS-Terra 11 µm brightness temperature greyscale image from Feb. 15, 2018 at 0545 UTC. Notice leads appear as bright (warm) features relative to the darker (colder) ice and clouds. [Granule projection onto a 1 km Equal-Area Scalable Earth Grid version 2 (EASE2-Grid). The region shown is north of 65° N, with the North Pole in the center of the map.] Credit: Jay Hoffman, CIMSS
“With the VIIRS finer spatial resolution, we thought that maybe we would see more leads than MODIS. But sometimes it detects more, sometimes it doesn’t. That’s part of what we’re trying to figure out,” says Ackerman.
Hoffman explains that part of the problem is cloud artifacts producing more noise, which affects the algorithm’s ability to accurately detect leads.
Clouds pose a unique problem in their research. Not only do leads play a role in cloud formation, those clouds can then obscure the leads, making them undetectable. Or alternatively, the clouds may be semi-transparent and leads can sometimes be detectable through thin clouds. Then the question is how to utilize a cloud mask — to prevent false lead detections primarily along cloud edges — while also minimizing lead detection omissions in the presence of thin clouds. The solution lies in choosing the best cloud mask, a critical step in adapting the algorithm for VIIRS.
While they continue their algorithm work, the team is already considering the questions they would like to answer when analyzing the data on sea ice leads and possible trends over time. First and foremost on their minds is how leads are changing with respect to thinning Arctic sea ice.
“One of the first hypotheses we had was that with decreasing sea ice we would see more leads. But we really haven’t found that to be true,” says Hoffmann. “One of the trends that we have detected is that we are finding fewer leads. But we haven’t been able to say with any statistical confidence that leads are decreasing. It might be that leads are becoming more numerous but more difficult to detect.”
Beyond quantifying the number of leads, future trend analyses include investigating possible correlations with changes in sea ice leads. For example, are the characteristics of sea ice leads such as width, orientation, and area changing in response to less and thinner sea ice? Similarly, the team plans to examine measurements of wind direction and strength and ocean current, looking for patterns suggesting potential connections.
Pattern recognition is another area of future research. With more than 15 years of sea ice leads products using MODIS data, the team is investigating whether machine learning could be used to detect leads in VIIRS data.
“The idea is we could use our archive of MODIS results — knowing what leads should look like — and if we see that same object shape in VIIRS, can we use a machine to tell us that that’s a detection?” says Hoffman.
As Key mentions, their team is far from the only group interested in studying this special area of ocean-atmosphere dynamics. Changing trends in the Arctic, and with regard to sea ice in particular, are indeed a hot topic. And yet, as Liu adds, their current work owes a debt to research begun several decades ago.
“Jeff [Key] did this 30 years ago, and this subject slept for almost 25 years. In recent years, people are starting to look at leads more … in observation, detection, and modeling,” says Liu.
This work was supported by NASA. To learn more about the algorithm, please read their paper “The Detection and Characterization of Arctic Sea Ice Leads with Satellite Imagers” published in the journal Remote Sensing.