Tweets and machine learning – Helping the National Weather Service understand its social media impact
For more than a century, the National Weather Service has provided the US with local and national severe weather alerts. Changing with the times and new technologies, the NWS has reached its communities through newspaper, radio and television. More recently, the agency has added Twitter to its toolkit.
Through a flurry of daily postings, people are engaging with local NWS Weather Forecast Offices more than ever on Twitter. Each day, their likes, comments, retweets and tagging help to amplify weather information like impending snowfall or rainy conditions.

But what types of NWS tweets catch the public’s attention? SSEC data scientist Iain McConnell aims to answer this question by building a model using machine learning that will be able to determine the effectiveness of an NWS tweet. This will help NWS WFOs craft more effective tweets, increase their reach and ultimately help save lives and protect property.
“Public attention is really fickle,” says McConnell. “What drives it isn’t necessarily the content of the tweet at all. It is hard to pinpoint what exactly spurs engagement.”
McConnell describes the different factors that play into a tweet’s engagement. One is the content itself: whether or not it includes a video, a photo, and the length and content of the message. However, McConnell has found no clear pattern with these factors alone.
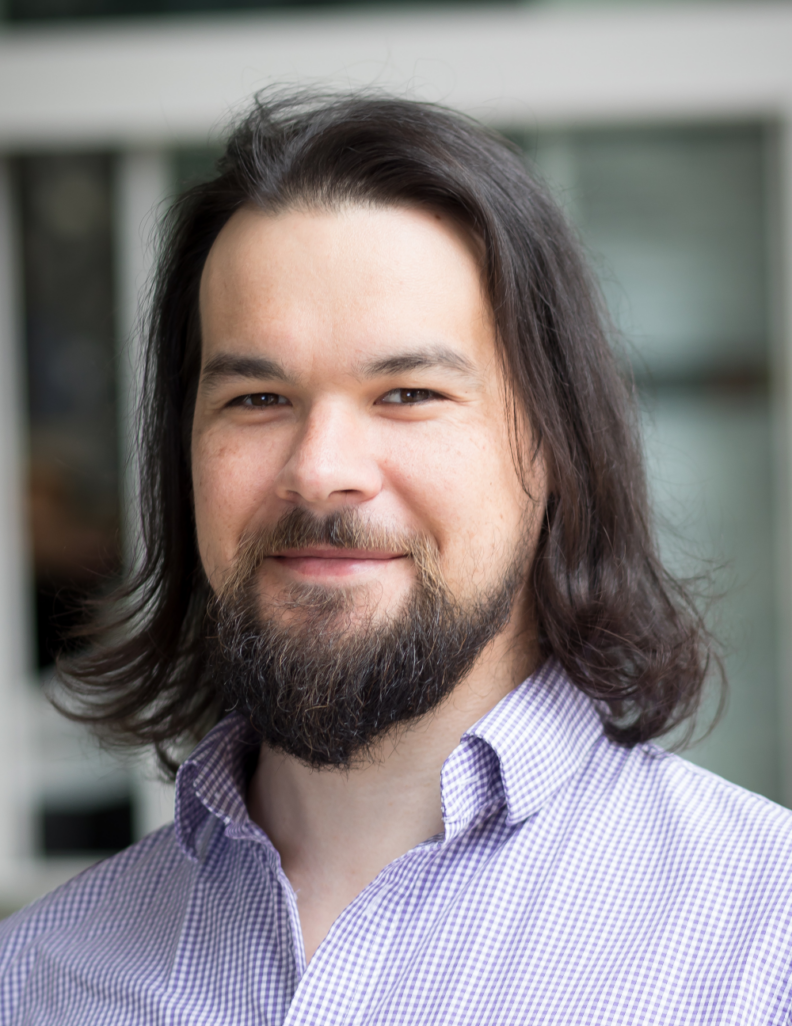
Credit: Iain McConnelle
McConnell says the environment at the time of the tweet, both social and atmospheric, influences engagement. If there is a big news story, scandal or trend that draws users to Twitter, an NWS tweet might get more attention as a result. Severe weather could also cause people to specifically look at the NWS Twitter accounts for updates and information.
Other factors, such as who interacts with the tweet, also matter. If a Twitter user with a large following interacts with an NWS tweet, it can bring that information to new audiences by way of a trusted source, thereby increasing the post’s engagement.
“In general, the majority of Twitter users are passive,” says McConnell. “Roughly 10 percent of users are ‘super users’ who tweet a lot, and may have more followers than most. They can be signal enhancers for other users, which adds another layer of complication to understanding attention and engagement.”
Currently, McConnell’s project is focused on the Milwaukee NWS station’s Twitter account, @NWSMilwaukee. His model is using one year of tweets from this account. To train the model, McConnell defines a popular tweet as having 10 or more likes. He explains that the definition of a popular tweet will be different for each WFO. Some offices will have higher engagement because they cover a larger metropolitan area. Other offices may have more engagement due to the type of weather that region experiences. For example, regions with more frequent supercells and tornadoes may attract more attention, including Norman, OK, Kansas City, MO, and Birmingham, AL.
According to a study in Weather, Climate, and Society by Eachus and Keim, there are now people that use Twitter as their primary weather information source. McConnell is studying the demographics, or characteristics, of this group to determine who makes up the NWS Twitter audience as a whole. The more we know about a group’s demographics, like age, education, or previous interest in weather, the more researchers can assess their likelihood of engaging with NWS tweets.
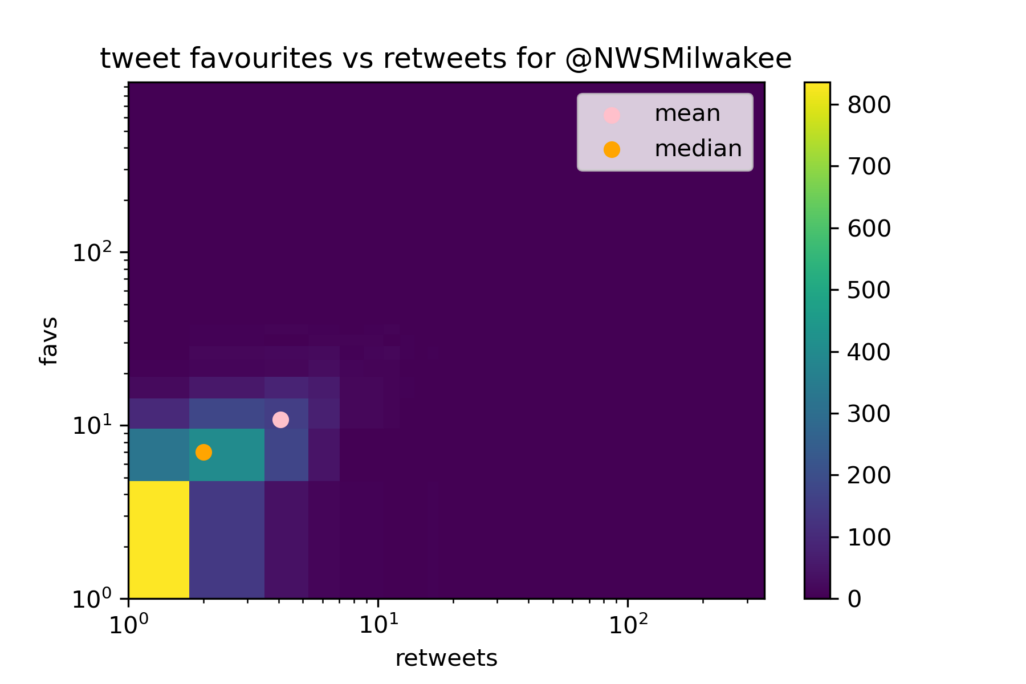
Using the model to answer these questions; however, could introduce biases in the analysis. McConnell cautions that focusing on specific demographics might leave out many people who are not currently part of the NWS Twitter audience. He wants the model to be able to capture that group while increasing public attention for reliable weather information.
Ultimately, McConnell’s goal is to enable and empower NWS forecasters to communicate more effectively on Twitter and to understand what makes someone want to engage with their tweets. At the same time, the public can learn about the benefits of more timely communications from their local weather stations. Although it is not part of the current project, this tool may help people stay informed about local weather, and may help them make better decisions as they respond to severe weather alerts.
Future goals of the project include using a larger historical dataset from the Milwaukee station and adding more NWS Twitter accounts to the model. Incorporating the time of the tweet, the weather at the time of the tweet and the social media environment into the model may yield better information that the NWS can use to reach even more people with important weather news or alerts.
This work is currently unfunded.