Hazardous Weather Testbed
Each year in May the National Oceanic and Atmospheric Administration Storm Prediction Center hosts the Hazardous Weather Testbed, an event that pairs researchers who are developing new forecast tools with forecasters who test them under real weather conditions. This unique forum helps to migrate promising applications from the testing environment to the operational environment with the National Weather Service to improve and deliver more accurate and timely severe weather forecasts.
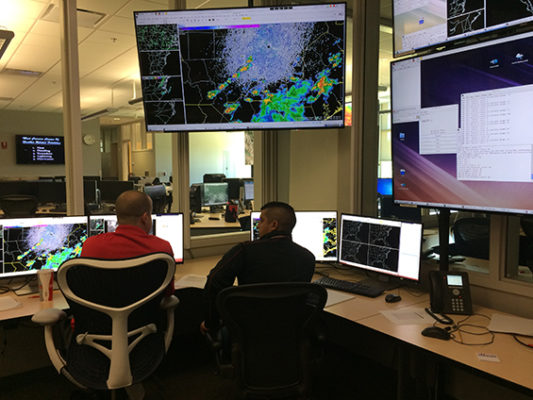
Credit: Justin Sieglaff, CIMSS.
For nearly a decade, scientists at the University of Wisconsin-Madison Space Science and Engineering Center and the Cooperative Institute for Meteorological Satellite Studies have participated in the HWT and this year is no different.
“It’s a very efficient and effective way to drive development of a product so that it is more operationally useful,” says CIMSS scientist and CIMSS Satellite Blog contributor Scott Lindstrom. “Scientists and developers don’t often have the opportunity to sit over the shoulder of a forecaster as they use tools and it provides a chance for forecasters to tell researchers what works and what needs to be improved.”
For example, feedback on the NOAA-CIMSS ProbSevere3 statistical model – one of three UW products being tested this year – gathered during the HWT is helping researchers improve the model’s assessment of developing storms: improvements that can increase the lead time for severe weather warnings. An earlier version of ProbSevere has been used operationally by National Weather Service offices since 2017, following years of testing within the HWT.
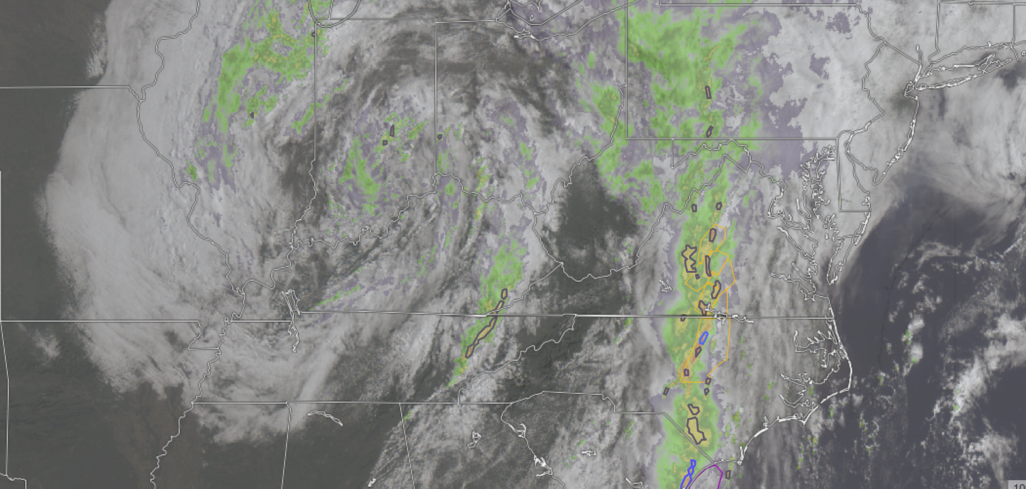
The other two CIMSS products under review include the ProbeSevere LightningCast model and the Polar Hyperspectral Sounding modeling system. The LightningCast is a machine learning model that uses visible, near-infrared, and long-wave infrared data from the Advanced Baseline Imager on GOES-16 and GOES-17 weather satellites to predict the probability of lightning in the next 60 minutes.
By predicting where lightning is most likely to occur in the future, forecasters are able to provide outdoor event managers , for example, with advanced notice that enables people to take shelter prior to the onset of lightning.
The Polar Hyperspectral Sounding modeling system incorporates thermodynamic information from hyperspectral soundings that are measured by instruments on polar orbiting satellites such as Suomi NPP and NOAA-20. The information is helping forecasters assess developing winds ahead of severe weather and understand the atmospheric conditions in and around clouds.
“Our partnership with NOAA is enabling us to develop and provide the best weather tools to meet forecasting needs to protect and serve the public,” says Lindstrom.
This work is supported by NOAA